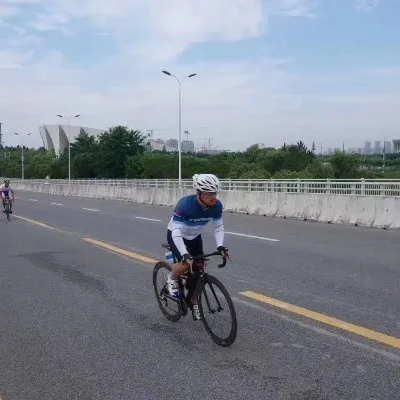
Lanli|蓝犁道人|Jan 30, 2025 19:07
This article is worth reading, with the following key points:
The breakthrough of AlphaGo lies in the fact that it does not require human correction, but rather plays against itself, giving rewards/punishments to train AI, which allows AlphaGo to surpass human chess players.
Previous generation AI: Speech recognition, image recognition (Hikvision), and autonomous driving are all previous generation AI algorithms, starting with AlphaGo defeating the human Go champion. The characteristic is that closed space games with clear rules and single goals are most suitable for reinforcement learning. The real world is an open space, with infinite possibilities at every step. There is no definite goal (such as "winning"), no clear basis for judging success or failure (such as occupying more areas of the chessboard), and the cost of trial and error is also high.
ChatGPT can be considered the next generation of AI: it generates intelligence in compression. Enable the model to generate intelligence in predicting the next word, then fine tune it through supervision to learn human question answering patterns, and finally use RLFH (Human Feedback Learning) to output answers that match human preferences.
What do people with CloseAI believe in? The group of people who firmly believe that compression is intelligence believe that as long as larger amounts of high-quality data are used and larger parameter models are trained on larger GPU clusters, greater intelligence can be generated. ChatGPT was born under this belief.
The problem of pre training hitting walls: Although the model volume has increased by 10 times, we are no longer able to obtain high-quality data that is 10 times larger than it is now. The rumors of GPT-5 not being released and domestic large model manufacturers not conducting pre training are all related to this issue.
Reasoning model: Using reinforcement learning (RL) to train the model's thought chain has become a new consensus for everyone. This training greatly improves the performance of certain specific, objectively measurable tasks such as mathematics and coding. It needs to start with a regular pre trained model and use reinforcement learning to train the reasoning thought chain in the second stage, which is called the Reasoning model. The o1 model released by CloseAI in September 2024 and the subsequent o3 model are both Reasoning models. Human feedback is no longer important, as it can automatically evaluate the results of each step of thinking and provide rewards/punishments.
DeepSeek's pure reinforcement learning model, named R1 Zero, is also a tribute to AlphaZero, the algorithm that surpasses the strongest player through self play without learning any game history. The training process of R1 Zero is completely independent of human intelligence, experience, and preferences, relying solely on RL to learn objective and measurable human truths, ultimately resulting in reasoning abilities far superior to all non reasoning models.
Distillation: usually refers to using a powerful model as a teacher, and using its output results as the learning object of a student model with smaller parameters and poorer performance, in order to make the student model stronger. For example, the R1 model can be used to distill LLama-70B, and the performance of the distilled student model is almost always worse than that of the teacher model, but the R1 model performs better than o1 in some indicators. Therefore, it is very foolish to say that R1 distills from o1.
Share To
Timeline
HotFlash
APP
X
Telegram
CopyLink