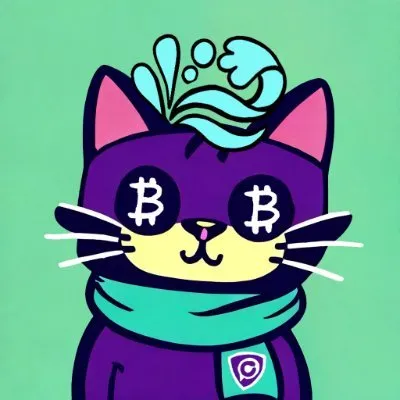
Lux(λ) |光尘|空灵|GEB|Apr 04, 2025 23:17
Adaptive Nonlinear Complex System Analysis from the Perspective of "Integration of Action and Knowledge": A Case Study of Bitcoin
Abstract: This article explores the applicability of the "integration of knowledge and action" concept in analyzing adaptive nonlinear complex systems, and compares its two main explanations. The traditional understanding of "knowledge is the beginning of action, and action is the completion of knowledge" focuses on deterministic planning, while the exploratory understanding of "action is the beginning of knowledge, and knowledge is the completion of action" is more in line with the characteristics of complex systems. The article takes Bitcoin as an example to demonstrate its special case as an adaptive nonlinear complex system, and elaborates on the process of Bitcoin miners calculating Nonce and the emergence of the longest chain consensus mechanism, demonstrating the exploratory logic of "integrating action and knowledge".
Keywords: integration of knowledge and action, adaptive nonlinear complex systems, Bitcoin, Emergence, exploration, certainty, uncertainty
1. Introduction
Adaptive nonlinear complex systems are widely present in the fields of nature and social sciences, characterized by nonlinear interactions between elements, emergent overall behavior, and the system's ability to adapt to environmental changes. Traditional analytical methods, often based on linear models and reductionism, often struggle when faced with such systems. This article introduces the ancient Chinese philosophical concept of "unity of knowledge and action" and explores its potential value in understanding and analyzing adaptive nonlinear complex systems.
2. Two interpretations of the concept of "unity of knowledge and action" and their philosophical implications
The concept of "unity of knowledge and action" is an important category in Chinese philosophy, particularly occupying a central position in Wang Yangming's philosophy of mind during the Ming Dynasty. However, there are two main interpretations of the order and interaction between "knowledge" and "action":
2.1 "Knowledge precedes action": This explanation emphasizes the guiding role of knowledge, theory, or plan in action. Action is based on existing knowledge frameworks, with the aim of verifying, implementing, or improving this knowledge. This viewpoint is consistent with the emphasis on theoretical construction and experimental verification in Western philosophy's rationalism and scientific methods, as well as the logic of deterministic system analysis, which determines outputs through clear rules and input predictions.
2.2 "Action precedes Knowledge": This explanation places action at the forefront of knowledge generation. Knowledge and understanding are not entirely a priori or predetermined, but gradually emerge and form through practice, exploration, and trial and error. The data, experience, and feedback generated by actions are the sources of knowledge, and the knowledge formed in turn guides future actions, forming a dynamic feedback loop. This viewpoint is more in line with the understanding of uncertain and complex systems, emphasizing the discovery of patterns through practice in the absence of complete prior knowledge.
3. Characteristics and analytical challenges of adaptive nonlinear complex systems
Adaptive nonlinear complex systems have the following key characteristics that make it difficult to fully predict and control them using traditional deterministic methods:
Nonlinear: The interaction between elements exhibits nonlinear characteristics, and small disturbances may cause drastic changes in the system state.
Complexity: The system contains a large number of interrelated elements, and the overall behavior is the result of the complex interaction of these elements.
Adaptability: The system is able to adjust and evolve based on changes in internal state and external environment, demonstrating dynamic adaptability.
Emergence: The macroscopic behavior and characteristics of a system are not simply the superposition of its microscopic components, but rather the spontaneous generation of new patterns and organizations through the interaction between elements.
These characteristics determine that the analysis of adaptive nonlinear complex systems cannot rely solely on pre constructed static models and determined rules, but needs to pay more attention to observing the behavior and discovering patterns of the system during its dynamic evolution process.
4. Bitcoin: A Case Study of an Adaptive Nonlinear Complex System
Bitcoin, as a decentralized digital cryptocurrency system, has typical characteristics of adaptive nonlinear complex systems:
Nonlinear: The price fluctuations of Bitcoin are influenced by various nonlinear factors such as market sentiment, speculative behavior, and regulatory policies. Network congestion and transaction costs also exhibit non-linear changes.
Complexity: The Bitcoin network consists of tens of thousands of nodes and miners distributed globally, whose behavior is driven by complex interactions of economic incentives, technological constraints, and consensus rules.
Adaptability: The Bitcoin protocol evolves through community consensus (although the process is cautious), with mining difficulty dynamically adjusted based on network computing power, and user behavior adapted to market conditions and technological developments.
Emergence: The consensus mechanism of Bitcoin's longest chain is not pre designed and enforced by centralized authorities, but emerged through the independent computation of Proof of Work by countless miners and its propagation in the network.
5. "Integration of Action and Knowledge" in Bitcoin Mining: The Emergence of Exploratory Knowledge
The mining process of Bitcoin, especially the behavior of miners constantly trying to calculate Nonce that meets the difficulty target, perfectly interprets the logic of "action is the beginning of knowledge, and knowledge is the achievement of action":
'Action is the beginning of knowledge': Miners do not know which specific Nonce value will generate a hash value that meets the difficulty requirements when performing hash calculations. They can only calculate by continuously trying different Nonce values ("rows"). Every hash calculation is a random exploration of the solution space of the Pang problem. At this stage, 'action' is the only way to discover potentially effective Nonce, prior to any definite 'knowledge'.
Knowledge is action ": When a miner successfully finds a Nonce that meets the difficulty target and combines it with the transaction data to be packaged into a new block to broadcast to the network, this block is verified by other nodes and ultimately added to the blockchain. The successful addition of this new block marks the update and extension of the blockchain state, and new 'knowledge' is thus generated. The history and consensus of the entire Bitcoin network have been gradually constructed and extended through the exploratory actions of countless miners. The longest chain consensus rule is not perfectly "known" and designed in advance, but an effective mechanism that emerges during the continuous "behavior" and verification process of network nodes. The behavior of miners follows economic incentives (obtaining block rewards), but their specific computational attempts are random and exploratory, ultimately resulting in the longest chain that maintains network security and consistency.
6. Conclusion
For adaptive nonlinear complex systems, relying solely on pre-set knowledge and determined rules for analysis often has limitations. The idea of "action is the beginning of knowledge, and knowledge is the completion of action" provides a more adaptable analytical framework. It emphasizes acquiring knowledge through active practice and exploration in the face of uncertainty and complexity, and guiding the evolution of the system through emerging patterns in practice. As a typical adaptive nonlinear complex system, Bitcoin's mining process and the formation of the longest chain consensus mechanism vividly demonstrate the effectiveness of this exploratory "integration of action and knowledge" logic. Future research can further explore how to combine this philosophical thinking with the methodology of modern complex systems science to gain a deeper understanding and mastery of the behavior of complex systems.
Share To
Timeline
HotFlash
APP
X
Telegram
CopyLink